INSIGHT
EVOLVING FROM MUSCLE TO COGNITION: AI IN 2019 AND BEYOND
Download the PDF
Sean Gourley, CEO, Primer
Robert Tsang, Director, Client Insights & Solutions, Financial Institutions Group, ANZ
October 2018
________
AS HISTORY HAS SHOWN, ONLY A HANDFUL OF TECHNOLOGY TRENDS HAVE TRUE STAYING POWER. MANY INNOVATIONS – SOME SIGNIFICANT, SOME NOVEL – EVENTUALLY FADE FROM THE SCENE. BUT LOOKING TOWARDS 2019, ONE TREND LIKELY TO ENDURE IS THE GROWING IMPACT OF ARTIFICIAL INTELLIGENCE (AI) AND MACHINE LEARNING ON FINANCIAL INSTITUTIONS IN AREAS SUCH AS CUSTOMER EXPERIENCE, RESEARCH AND ADVISORY, OPERATIONAL PROCESSES, COMPLIANCE AND RISK MANAGEMENT.
The big change now is that we’re moving rapidly from a world in which machines automate heavy lifting and ‘muscle work,’ to one in which algorithms conduct tasks requiring some level of cognitive skills – much like humans.
Given these changes, over the next few years financial institutions will apply an increasingly diverse range of algorithms to a broader set of service offerings, unleashing unimaginable efficiency gains, although these gains will be tempered by the inevitable upheaval from some jobs becoming redundant.
As ever, changes will occur at a breath-taking pace. For evidence of this pace, look no further than the recent history of Nokia and Apple. In 2007, Fortune magazine crowned the then telecommunications giant Nokia “the king of cell phones” on its front cover; meanwhile in California, Apple released the first generation iPhone. Come 2018, the market value of Apple crossed the US$1 trillion mark, iPhones are one of the dominant icons of the tech revolution and Microsoft (which acquired Nokia in 2014) has written off most of the acquisition price it paid for Nokia.
A key lesson from this is that the future, especially when it comes to technological advancements, is rarely linear, so it would be prudent not to take all of today’s extrapolations into the future as a given. It took only eleven years for Nokia to go from the undisputed king of mobile, to being completely usurped by new entrants like Apple and Android phone makers.
MORE INVESTMENT, MORE ALGORITHMS
The key ingredients of AI innovations are (1) data; (2) computing power; and (3) algorithms. Data in many ways is the hardest part as it tends to be inherently flawed and based on the structural biases from the past. The need for better, and cleaner data and more computing power requires significant amounts of capital.
To get a sense of where we’re headed in the next few years, it’s helpful to first look at the numbers.
The World Economic Forum, in a recent publication, estimates at least USD $58 billion in global AI investment by 2021, including USD $10 billion from financial institutions. These estimates represent a 48 percent CAGR in global cross-sector AI investment over that time-period.
As investment increases, the range of algorithms used for commercial purposes will also expand accordingly. There are two major groups of algorithms to consider: ‘supervised’ and ‘unsupervised.’ Supervised means input and output variables are clearly defined; the algorithms map patterns to make predictions within predetermined parameters. For unsupervised algorithms, outcomes are not clearly defined; algorithms are left on their own to decipher and decode trends in large data sets.
The following table sets out the different types of algorithms and some sample real life applications.
TABLE 1:
Examples of “Supervised” vs. “Unsupervised” algorithms

Source: Primer, ANZ Analysis
_________________________________________________________________________________________________________
HOW CAN FINANCIAL INSTITUTIONS APPLY THESE ALGORITHMS OVER THE NEXT FEW YEARS?
_________________________________________________________________________________________________________
NEW EFFICIENCIES FOR FINANCIAL INSTITUTIONS
1. Feedback mapping
One place to start is client – or for retail banks, customer – feedback mapping. In the digital era customers often express their views of a bank’s service on a range of social media platforms and websites. Given its volume, banks have no way of aggregating or analysing this data, and its value is lost.
Banks can use a range of algorithms, e.g. clustering, to draw insights from this data, such as how to improve service in a certain area, or to identify how certain customer or client segments experience or perceive a particular service category. This could not only drive better customer engagement, but could uncover new revenue growth opportunities as well.
2. IT trouble shooting assistance
Another area of AI application, particularly using Neural Networks, is the automation of customer assistance, for example in online banking services. Robots can solve customer problems by analysing user-specific problems and configuring tailor-made solutions.
3. Equities or Investment Research
Yet another area ripe for AI-driven innovation is in the area of equities or Investment Research. Millions of data points can feed into the assessment of a particular security – not only balance sheets and profitability ratios, but also social media feeds, conference calls, public statements by senior executives, and historical circumstances and context.
Given the volume of information, it is very challenging for a human analyst to distil such a large volume of data into a succinct conclusion in a short time. But in the blink of an eye, an Artificial Neural Network algorithm with Natural Language Understanding can synthesise millions of data points and generate inferences and conclusions in a report much like the one on Starbucks below.
4. Portfolio construction and trading strategies
Related to the above, Neural Network algorithms can also construct portfolios, including ETFs and other tradeable securities based on a series of risk-based inputs and commands. For example, “Companies with an elevated competition risk in China” generates the outputs shown in figure 4. AI can do this much quicker – and with a much higher degree of accuracy – than any human, even the brightest minds among us.
Where human brains are limited by capacity and processing speed, machines overcome this limitation by their ability to synthesise large amounts of data in very short time frames. This incredible speed differential is where AI and machine learning can complement human cognitive capabilities.
_________________________________________________________________________________________________________
“FINANCIAL INSTITUTIONS REQUIRE TAILORED AND RELEVANT PRODUCTS, AN EFFICIENT BACK OFFICE AND EFFECTIVE USE OF DATA TO DELIVER GREAT OUTCOMES TO CUSTOMERS AND SHAREHOLDERS. WITH APPROPRIATE STRATEGY AND EXECUTION, INNOVATIVE FINANCIAL INSTITUTIONS WILL BE ABLE TO BEST ADDRESS THESE CHALLENGES USING AI. THOSE THAT ARE UNABLE TO PROVIDE THESE SERVICES AND NAVIGATE THE CHANGING LANDSCAPE RISK BECOMING IRRELEVANT.”
RICHARD PRIDE, FINTECH LEAD, HONG KONG, FIG, ANZ
_________________________________________________________________________________________________________
HEEDING THE LESSONS OF AI MISHAPS
While it is natural to be excited about new AI developments,
it is also wise to temper that excitement with a sober view of how AI can cause damage if it isn’t managed properly. For example, high frequency trading algorithms can cause massive losses due to amplification of errors in machine time. We saw this play out in real life in 2012 when an AI-driven malfunction caused Knight Capital to incur USD $440 million in trading losses in mere minutes, leading to the firm’s sudden and irreversible collapse.
Then there are lessons of inherent bias in historical data, which do not capture any feedback loop of how the world would adapt to information, and the consequent impact on an AI algorithm’s accuracy: e.g. Google’s Google Flu Trend (GFT) project, which attempted to aggregate ‘flu’ search data to calculate rates of flu infections. The basic premise was that people search for ‘flu’ when they are sick. While the algorithm performed well during the period 2009-2011, from 2011 to 2013, GFT persistently overestimated flu infection rates, at the highs more than double the estimates by the Centers of Disease Control and Prevention. This was partly due to the algorithm’s failure to account for changes in search behavior over time combined with new health based add-ons in the Google search engine which made some disease related search terms more prevalent.1
As Weiner’s Law puts it: Automation will routinely tidy up ordinary messes but will occasionally create an extraordinary mess.
WILL HUMANS BECOME REDUNDANT?
As with any significant trend – especially one straight out of the pages of science fiction – a mixture of hype and hysteria surrounds AI evolution. But there’s good news for professionals worried about being displaced by robots, and that is: As prediction and data analysis become cheaper, the value of human judgement increases.
The hope for humans in this new paradigm is in more nuanced and creative processes; circumstances in which the combination of AI and human abilities produces superior results.
For example, between 1991-2003 a human-AI partnership demonstrated a 16% improvement in weather prediction accuracy over a pure machine environment.2 A similar outcome is seen in the area of tumor detection: in one such study, the combination of human and AI intelligence saw a 99.5% success rate, compared to 92.5% for AI alone, and 96.6% for only human.3
_________________________________________________________________________________________________________
“GIVEN THE CHANGES AND CHALLENGES THAT AI WILL BRING, THERE WILL BE OPPORTUNITIES FOR COLLABORATION BETWEEN FINANCIAL INSTITUTIONS AND USERS TO JOINTLY SHAPE THE FUTURE. ANZ IS OPEN TO EXPLORING THESE OPPORTUNITIES WITH OUR CLIENTS AND NEW ENTRANTS.”
SHANE MARSH, HEAD OF SINGAPORE, FIG, ANZ
_________________________________________________________________________________________________________
OPPORTUNITIES AHEAD
The opportunities seem endless for more effective AI and human partnerships as we look into the next decade.
As a start, it is obvious financial institutions could benefit from image recognition applications that improve Security IDs and ease the burden of insurance claims. Meanwhile, AI natural language understanding could improve the accuracy and depth of investment research and trading, and also unleash new efficiencies within the legal and compliance disciplines.
The sheer potential of such innovations shows that rather than worry about some kind of hostile robot takeover, we should see AI as reliable partner in the building of a more sustainable human future.
1. https://www.wired.com/2015/10/can-learn-epic-failure-google-flu-trends/
2. Novak, D.R.; Bailey, C.; Brill, K.F.; Burke, P.; Hogsett, W.A.; Rausch, R.; Schichtel, M. Precipitation and Temperature Forecast Performance at the Weather Prediction Center. Weather Forecast. 2014, 29, 489–504
3 Cui W, Wang Y, Fan Y, Feng Y, Lei T (2013) Localized FCM clustering with spatial information for medical image segmentation and bias field estimation. Int J Biomed Imaging 2013, Article ID 930301
RELATED INSIGHTS AND RESEARCH
insight
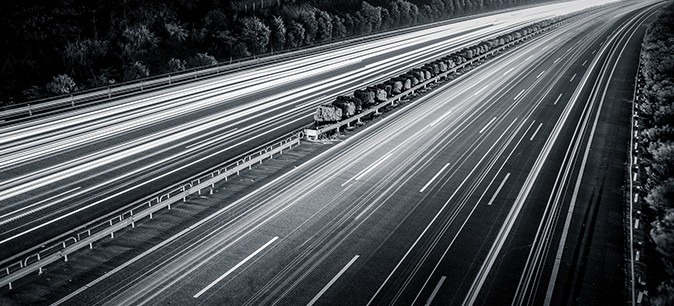
ASX Listed Investment Vehicles Are Changing Fast
While listed investment vehicles (LIVs) have been around for a long time, 2017 was a watershed year in IPOs... The size of these LIV raisings have led to evaluated interest in the sector by a range of local, regional and global players.
insight
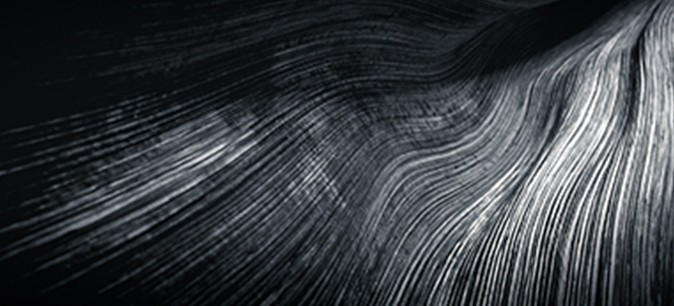
To Hedge Or Not To Hedge: That Is The Question
As QE comes to an end and inflation expectations pick up in the US, it's clear that the world of persistently low USD interest rates is disappearing.
insight
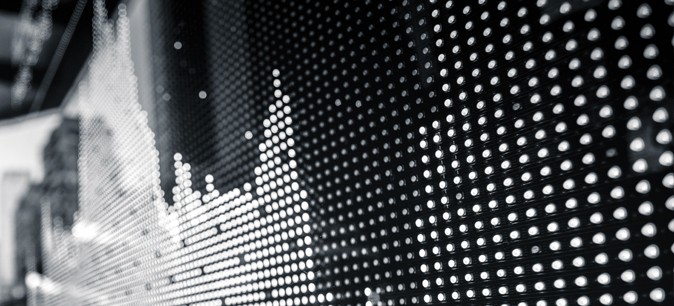
The Evolution of FX Risk Management Funds
History shows ignoring FX shifts can prove costly for fund managers – and in 2018 that remains truer than ever, especially for sector-specific specialty funds and long-dated funds that traditionally rely on forwards to manage FX risk.